Methodology
Disclaimer: The square footage information provided on this website is generated using advanced AI models, licensed data, and professional sources where applicable. While we make every effort to ensure accuracy, these measurements are estimates and may not be fully precise. We recommend verifying property details with official organizations, legal documents, or professional measurements for the most accurate and reliable information. Gnowise assumes no liability for any discrepancies or decisions made based on the information provided on this website.
Updated on Jan 1, 2025
At Gnowise, we prioritize transparency and accuracy in calculating living areas for properties across Canada. Our methodology leverages advanced satellite imagery, AI-powered models, and collaborations with professionals to ensure compliance with legal standards and deliver reliable results. By documenting our process comprehensively, we address ambiguities and minimize legal concerns while building trust with our clients.
1. Data Collection
1.1 High-Resolution Satellite Imagery
We utilize high-resolution satellite imagery to create Digital Surface Models (DSMs) and Digital Terrain Models (DTMs). These models enable the extraction of building features, including:
- Building footprint
- Rooflines and elevation
- Parcel layout
1.2 Publicly Available Datasets
Our AI models are trained on millions of high-quality, publicly available samples from carefully selected U.S. regions that mirror the urban and architectural characteristics of Canadian areas. The extensive and robust datasets ensure precise and accurate training, enabling our models to deliver reliable results.
1.3 Licensed and Professional Data
To ensure accuracy for condominiums and other complex structures, we collaborate with professionals to obtain verified data. This includes precise living area measurements, detailed floor plans, and legal documents, achieved through floorplan measurement, augmented reality (AR) smartphone applications, and photogrammetry. In some provinces like Quebec, we leverage direct figures from provincial official sources.
2. Data Processing
2.1 AI-Powered Modeling
Our proprietary AI models analyze satellite imagery and auxiliary data to calculate living areas. Key steps include:
- Feature Extraction: Identifying structural details such as rooflines, boundaries, and lot dimensions.
- Geometric Calculations: Estimating living areas using advanced techniques like triangulated irregular networks (TIN) applied to DSMs and DTMs.
- Volume and Area Derivation: Calculating total building volumes and converting them into living areas using empirically validated models.
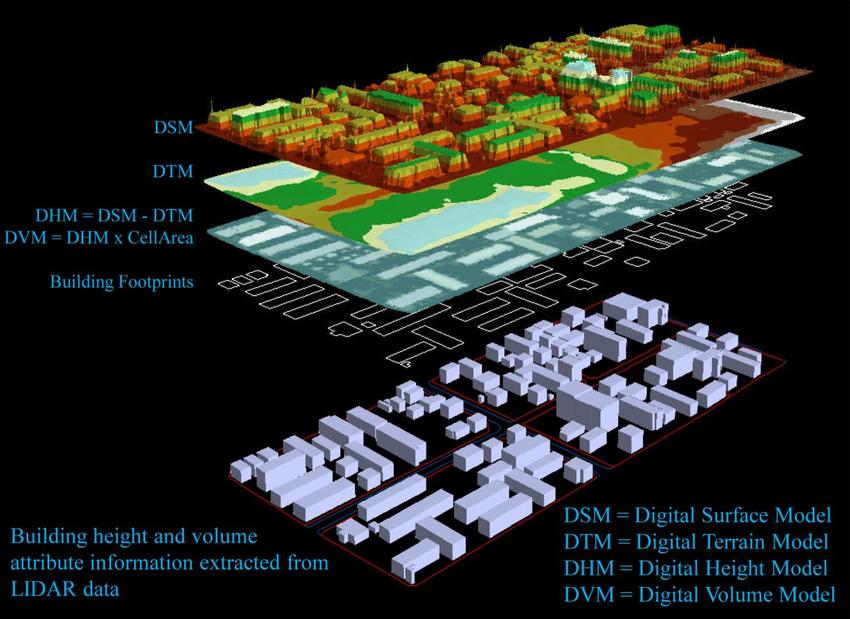
Illustration source: https://www.researchgate.net
2.2 Standardization
All collected data is processed into standardized formats to ensure consistency and comparability across different regions and property types.
3. Quality Control and Validation
3.1 Accuracy Assessment
Before deployment, our models undergo extensive testing against verified datasets. This includes comparison with licensed data and professional measurements to identify and resolve any discrepancies.
3.2 User Feedback Loop
We engage participating users to collect feedback on calculated living areas, including percentage differences from human-measured properties. This feedback refines our models and improves overall accuracy.
3.3 Regional Adjustments
Region-specific architectural characteristics and legal standards are incorporated into our models to ensure precise calculations. For instance, urban high-rises in Toronto are treated differently from rural properties in Ontario.
4. Testing and Best Practices
4.1 Diverse Sample Testing
Our AI models are rigorously tested on a diverse range of properties, ensuring reliability across various geographies and property types, from urban condos to suburban homes.
4.2 Established Best Practices
We adhere to best practices in AI modeling, including:
- We train our models using high-quality datasets from U.S. regions that closely mirror the architectural and urban characteristics of targeted areas in Canada.
- Regular recalibration of models with new data.
- Transparency in algorithmic assumptions and decision-making processes.
4.3 Scientific Validation
Our methodologies are grounded in peer-reviewed research, such as Lee & Li’s “AI-Based DSM and DTM Processing for Urban Building Volumes” and similar studies on volumetric analysis using satellite data.
5. Ensuring Transparency for Legal Compliance
Transparency is the cornerstone of our approach. To build trust and ensure compliance with legal standards, we:
- Share error margins and confidence intervals for AI-derived calculations.
- Clearly distinguish between AI-generated data, licensed data, and direct registry inputs.
- Provide detailed documentation of our methodology and regional practices.
In Ontario, where data primarily comes from AI models, we emphasize the robustness of our validation and user feedback systems. In some provinces like Quebec we rely on registry-provided data, ensuring legally compliant measurements.